Research Focus
Artificial Intelligence for Protein Diagnostics
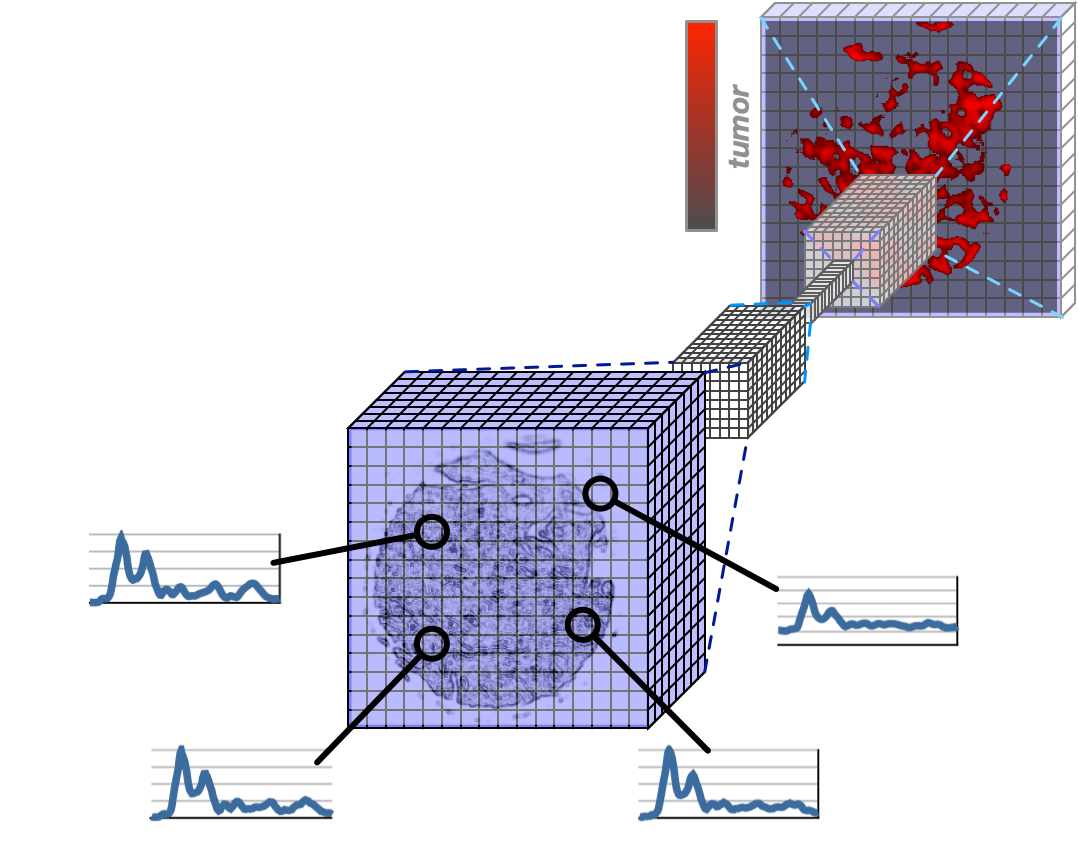
Within just a few years, artificial intelligence (AI) has taken an important role in medical research and is on the way to becoming an important diagnostic tool in many areas. For the molecular and analytical methods established in PRODI, AI is an important tool in order to fully exploit the potential of the approaches in protein diagnostics.
AI reveals disease patterns. The signatures of disease-specific molecular changes are often latent in biomedical data and are not directly accessible for diagnostic purposes. This is where artificial intelligence comes into play: If sufficiently large amounts of data are collected in clinical studies, the decisive patterns can be uncovered with techniques such as deep neural networks (or deep learning for short), which can be used to differentiate between subtypes of cancer.
Our research approach. The research focus in the competence area of bioinformatics lies in the establishment of AI techniques in order detect disease patterns and use them diagnostically. Our research approach is based on the development of AI methods with the aim of making the output of AI systems molecularly explainable.
Explainable artificial intelligence. In many applications, deep learning models can classify the disease status of samples and patients into predefined categories with very high accuracy. However, the underlying neural networks are inherently a black box: the neural network does not provide any explanation as to why the sample was assigned to a specific category. This leads to the field of explainable artificial intelligence, or XAI for short. In recent years, explainable artificial intelligence has become a research area within artificial intelligenc.
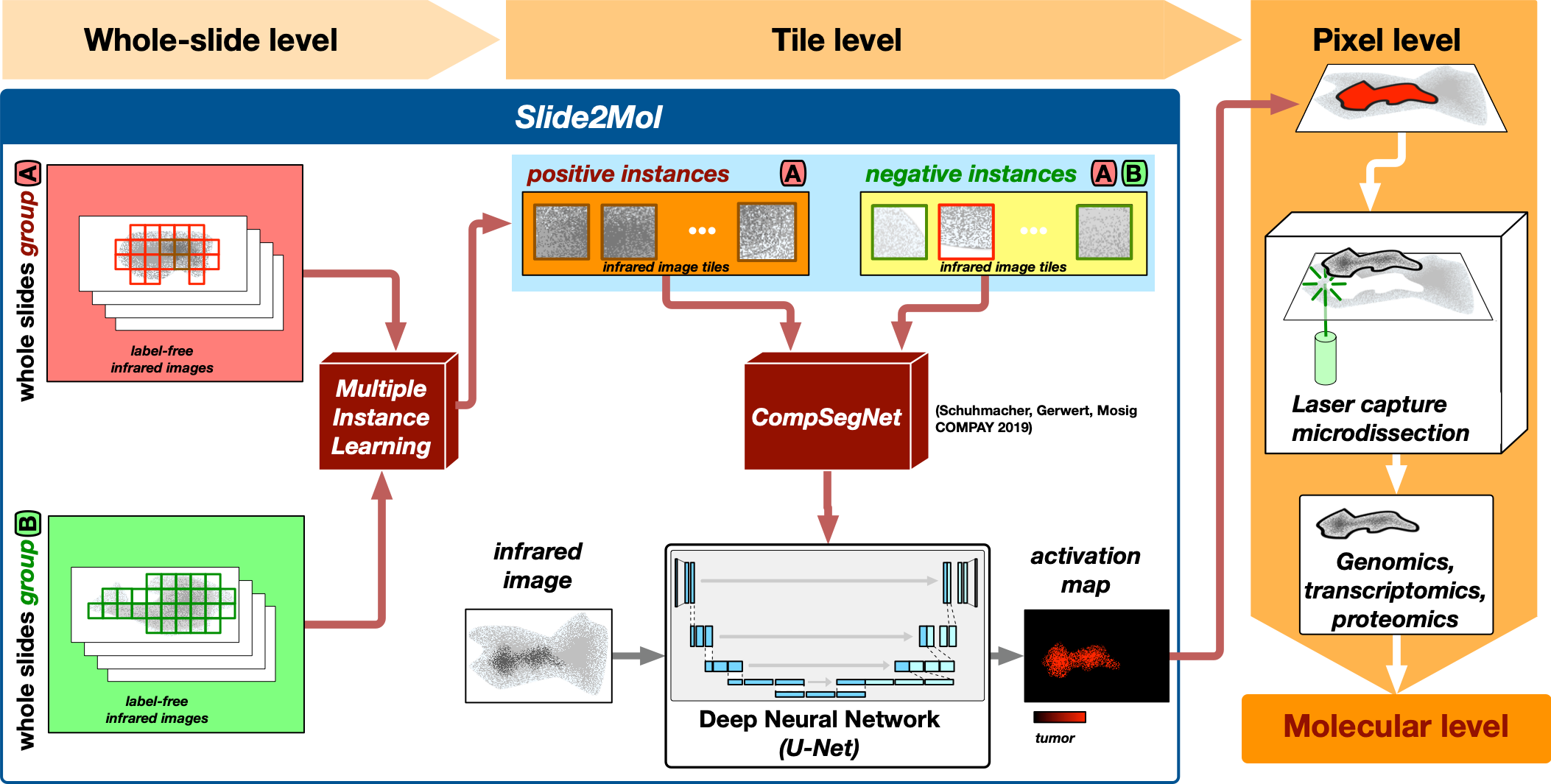
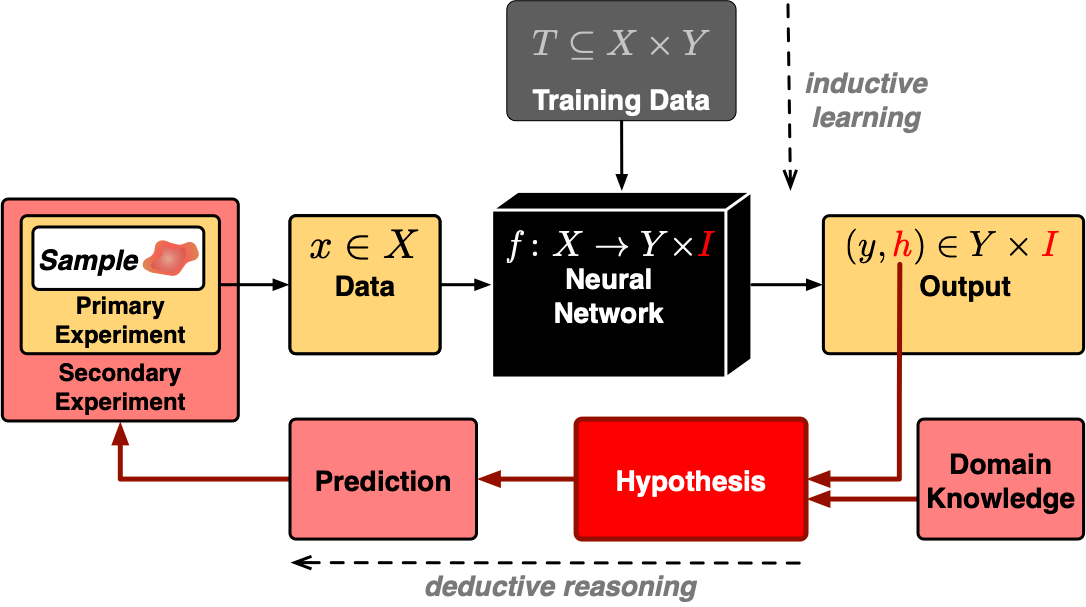
Molecularly explainable deep learning. The AI development in the competence area of bioinformatics is closely linked to experimental and molecular research in other competence areas of PRODI. This enables a dedicated research approach: The disease patterns localized by AI methods can be further characterized on a molecular level. This allows us to connect the output of AI methods with experimentally testable hypotheses. These hypotheses thus provide a molecular explanation of the output of AI systems. Because these explanations can be examined experimentally, they particularly fulfill the main goal of explainable artificial intelligence, namely to underpin trust in AI-based classification. This approach is particularly suitable for infrared IR) microscopy: Because IR microscopy works label-free, subsequent molecular characterizations can be carried out particularly easily.
Falsifiable explanations in machine learning. The approach of molecularly interpretable artificial intelligence follows a more general scheme of falsifiable explanations of machine learning systems. Central to this approach is a hypothesis that relates the classified sample to an output inferred by a machine learning system.