Method development
Bioinformatics
Workflows for biomarker candidate detection
The biostatistical workflow for the medical samples already begins with the planning of the proteomic studies. Before samples are taken, the necessary size of the entire study is coordinated with the scientists of all areas of competence in order to obtain statistically significant results later.
As soon as samples have been measured, the data evaluation workflow begins: first, the samples are analysed for their quality using quality control metrics in order to detect possible outliers as early as possible. The recording of the metrics is an automated process in which values are collected at the raw measurement data level as well as initial identification and quantification numbers of the samples.
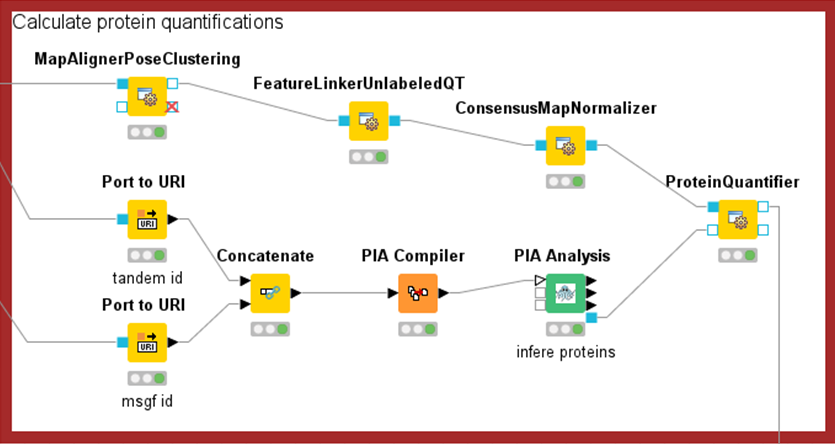
The measurement data are then evaluated by means of bioinformatic analyses. Here, the proteins of a sample must first be identified. In the case of mass spectrometry-based methods, the correct choice of protein sequence databases and threshold values is relevant for limiting false-positive results. In addition to identification, protein quantification is also performed. As with all high-throughput measurements, minor systematic measurement inaccuracies can occur in proteomics, which are compensated for with normalisation methods.
Once the data have been prepared appropriately, biomarker detection methods can be applied. Univariate statistical tests, but also clustering methods and machine learning methods are applied. Finally, the biomarker candidates are verified in silico using discriminant and ROC analyses. Candidates found in this way must then be validated by wetlab laboratory experiments.
Optimisation of methods and existing evaluation software
Bioinformatics methods are continuously being developed on all levels mentioned in the workflow. Often, it is also necessary to adapt an established method to the respective study in order to obtain high-quality results. New technical possibilities of mass spectrometers also pose new challenges. For example, the amount of data per measurement has continued to increase in the past. This has resulted in an efficient adaptation of the bioinformatics methods and infrastructure in order to be able to continue to use this data efficiently. But also due to the increasing accuracy of the measurement methods, older methods, e.g. for limiting the false-positive rate of peptide identifications, are partly no longer applicable, but instead completely new questions can be analysed bioinformatically.
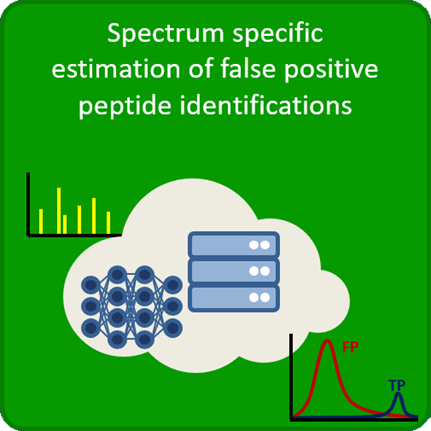
Figure 2: Optimisation of methods to limit the false positive rate
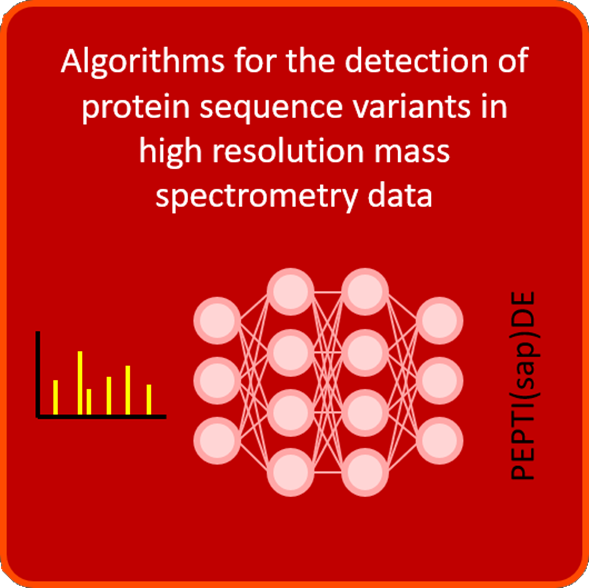
Deep Learning for the detection of protein variants
In the protein sequence databases usually used for the detection of proteins and peptides, only the canonical forms of the proteins are listed. Although many variants and mutations of proteins are already known through e.g. genome sequencing projects, these are rarely used for identification so far. However, many of these already annotated variants are specific to cancer and neurodegenerative diseases and are therefore of particular interest for research in the PRODI.
Bioinformatics is pursuing strategies to be able to detect these variants in mass spectrometric measurements. On the one hand, procedures are being developed to extract the large number of variants from the databases in a disease-specific manner. Here, the already mentioned improved approach for limiting false positive identifications must also be taken into account, as this is always particularly important when search spaces become especially large.
On the other hand, Deep Learning methods are applied to develop a more sensitive database search for spectra identification, as well as a method for de novo identification of spectra. For the latter, no protein sequence databases are necessary, but possible variants and mutations are only identified in a subsequent comparison to the database sequences. Both of the Deep Learning methods mentioned above not only help to detect previously undetectable disease-specific variants and mutations, but also to track down the underlying peptide sequences for mass spectra that were previously unidentifiable and are therefore counted among the “dark matter” of proteomics.